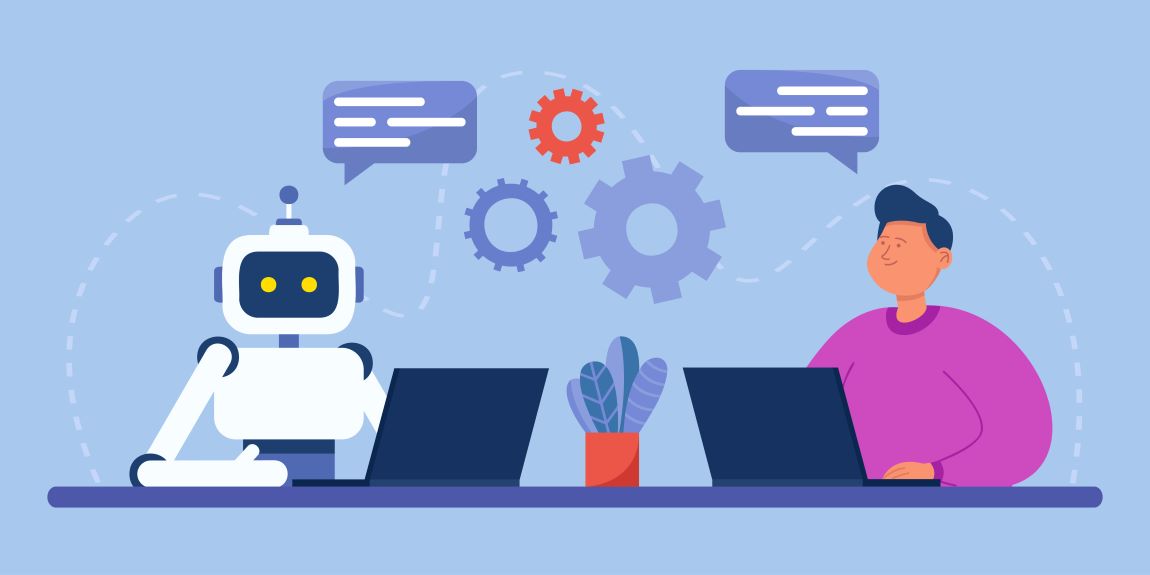
Unlocking the Future of Insurance with AI and ML
The insurance industry, known for its cautious approach, is on the brink of a major revolution. Artificial Intelligence (AI) and Machine Learning (ML) promise to streamline processes, enhance decision making and personalize customer interactions. However, integrating these technologies can come with its own set of challenges, like getting around risk-averse mindsets and legacy systems. So, how can insurance companies effectively adopt AI and ML to stay ahead of the curve?
Supervised, Unsupervised and Reinforcement Learning
To get the most out of ML, it’s important to understand its various forms – supervised, unsupervised and reinforcement learning. Of these forms, supervised learning currently offers the most benefits for businesses, including insurers, while clarity on uses for unsupervised and reinforcement learning in insurance is still emerging.
Supervised learning involves training algorithms on large sets of tagged and labeled data until the algorithms can recognize patterns in previously unseen data and even predict labels. This basic technique introduces opportunities for insurers to improve product personalization, risk assessment, claim severity prediction, fraud detection and customer lifetime value prediction — just to name a few.
What About Generative AI?
Lately, much of the common discourse surrounding AI is consumed by generative AI, or GenAI — a class of AI tools that can write text, draw images and produce videos. GenAI is the result of training ML models using a combination of supervised learning, unsupervised learning and reinforcement learning, and has gained prominence due to the human-rivaling content it can create.
To Build or Not to Build: Strategic AI Considerations
Jumping into the world of AI can feel like standing at a crossroads for many insurers. On one path, there are general-purpose AI tools, ready to use right off the shelf. These tools work across industries — not just in insurance — making them an easy pick for companies eager to get started with AI. Then, there’s a path marked with insurance-specific products, tailored to fit an insurer’s unique needs. Both options are straightforward to adopt, requiring minimal fuss to integrate into existing systems.
But the third path is a more involved and certainly less traveled: building custom AI models in-house. This requires investing in new skills, such as data engineering, data science, model engineering and ML/Ops. Not every company is ready to make this leap, leading to fractures in the industry.
Those who stick to the well-trodden paths of general-purpose tools or industry-specific products might find themselves moving faster at first, temporarily outperforming other insurers. However, insurers developing custom models will unlock true opportunity. Leveraging unique data and circumstances will eventually set those insurers apart, offering them the chance to not just participate in the AI revolution but lead it.
Navigating Explainability, Accuracy and Bias
While diving into the world of AI and ML opens a future full of potential, the path is not free of hurdles. One significant roadblock? Explainability. When it comes to decision-making, understanding why a decision is made can be just as important as the decision itself. AI models, particularly those based on ML, operate in highly complex ways that can obscure how they work. This brings up three major questions: are the models correct, do they perpetuate biases and are they fair.
At their core, ML models are statistical, so no matter how accurate they seem, there’s always a degree of uncertainty. They’re like weather forecasts for data — predictive yet not perfect. Current ML models can’t show their work either, adding to the uncertainty. Imagine solving a math problem but not being able to explain your steps. That’s where AI is currently. Because of this, those offering AI tools can only suggest a “safe zone” for their use without clear boundaries.
Bias in data is another issue. If the information fed into these models contains biases, the AI’s decisions will as well, continuing patterns of unfair treatment of disadvantaged groups. The silver lining? Unlike human biases, which are hard to identify and change, ML biases can be spotted and tweaked quickly. Navigating AI and ML’s future means wrestling with these challenges head-on, seeking clarity, fairness and correctness in a journey that’s just getting started.
Leading the AI Charge: The Role of Leadership
Navigating the AI landscape requires strong leadership coupled with technological expertise, leading to the creation of the Chief AI Officer (CAIO) role. This leader must have a deep understanding of AI capabilities and limitations, experience building AI solutions and a firm grasp of business challenges and opportunities to ensure AI initiatives align with business objectives and deliver real value.
Insurance technology leaders stand at the forefront of a significant shift. The journey toward integrating AI and ML is complex, but undeniably rewarding. By understanding the nuances of different AI disciplines, making strategic decisions on AI adoption, considering the unique challenges presented by these tools and emphasizing strong leadership, insurance leaders can unlock new opportunities and drive their company forward.
Keep the Conversation Going
What’s your take on integrating AI and ML into insurance? Are you leaning toward building your own solutions, or do you find value in off-the-shelf products? Share your thoughts and strategies with us. Let’s shape the future of insurance together. Reach out to Samir directly at sahmed@xby2.com.
Related Articles
-
Measuring digital transformation's impact can be affected by external factors but is possible when focusing on measurable outcomes.
Read More -
Analytics is becoming even more crucial for insurance, sparking a variety of perspectives on how to embed analytics into the industry.
Read More -
The long-term care insurance value chain can be revolutionized by consumer healthcare technology innovation and data ecosystems.
Read More