Proactive Care Management with Predictive Modeling
The possibilities with artificial intelligence (AI) are vast, yet healthcare payers and providers still struggle to determine how, when and where AI can make a true impact within their organization. Given the unique gaps and opportunities in chronic care management, predictive patient admission and care intervention may be the best places to begin.
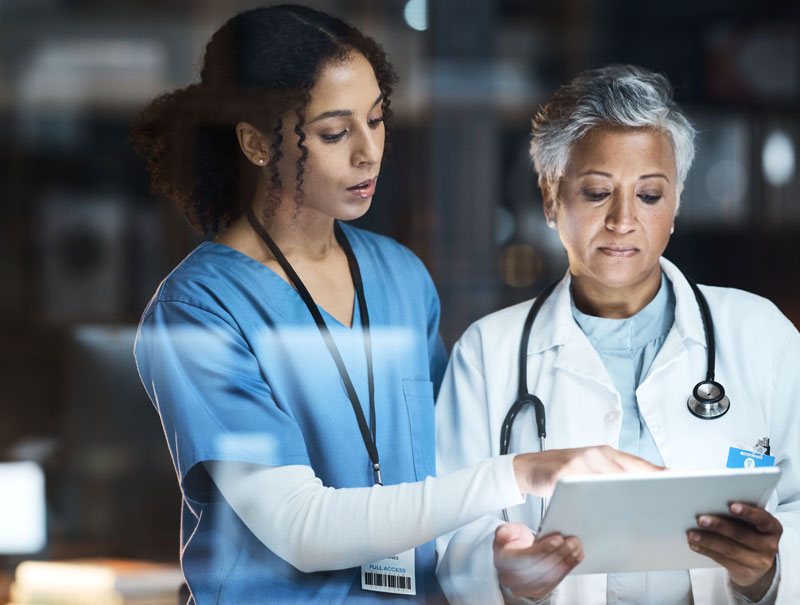
The Client
Health Insurance
Provider and Network
The Business Objective
A significant resource gap exists in care management, and healthcare is trying to keep up. With a high prevalence of chronic disease in the U.S., it’s challenging for healthcare organizations to, first, identify patients who need the most help managing their conditions and, second, intervene in ways that help avoid the life-impacting consequences of their diseases. Unfortunately, traditional methods of identifying at-risk patients aren’t tailored or standardized to ensure preventative measures are suggested early enough to be effective.
In 2018, a reported 52% of adults in the United States (129 million) suffered from chronic conditions, 68 million of whom suffered from more than one.¹ Due to the shortcomings of traditional patient selection methods, care managers are often overwhelmed by long lists of patients and left to make highly subjective decisions about patient care. Simultaneously, they’re expected to process large volumes of patient data, clinical notes and administrative claims. Their excessive workloads result in inconsistencies across large organizations as well as lower quality care decisions — which are influenced by the skill and experience of the care manager.
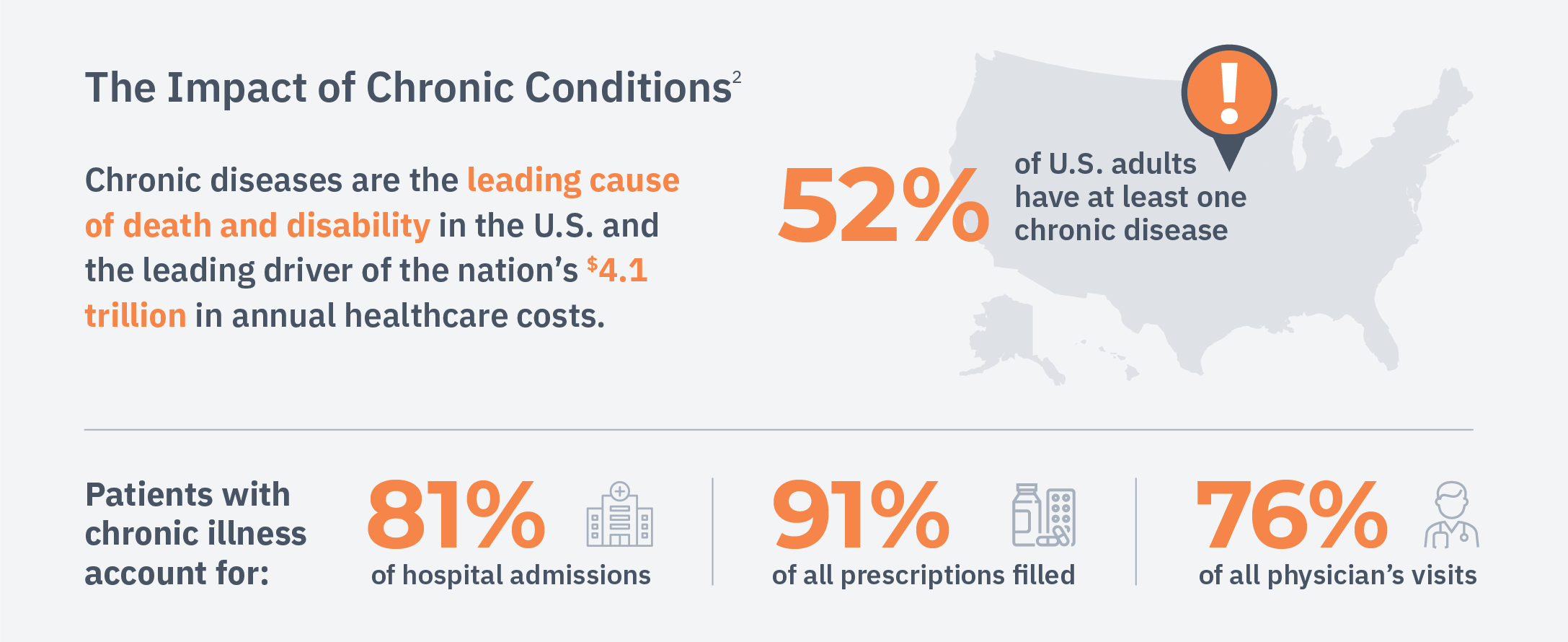
- Boersma P, Black LI, Ward BW. Prevalence of Multiple Chronic Conditions Among US Adults, 2018. Prev Chronic Dis 2020;17:200130. https://www.cdc.gov/pcd/issues/2020/20_0130.htm
- https://www.fightchronicdisease.org/sites/default/files/docs/GrowingCrisisofChronicDiseaseintheUSfactsheet_81009.pdf
The Business Challenges
AI has been used to help automate and standardize patient selection criteria, however traditional algorithms and models fall short. When early algorithms were designed, they relied upon data used primarily for reimbursement purposes, which didn’t always accurately reflect the health status of the patient, even with a complete patient history. These techniques could only take in highly structured patient data, such as ICD and CPT codes, and required precise and complete medical coding of the patient’s history. As a result, minor coding inaccuracies or gaps in data could cause these algorithms to produce inaccurate risk scores.
Additionally, the traditional models used for risk stratification were developed to answer a specific question from a fixed perspective. The models are not agile; when used in the wrong context, they are rendered crude tools that can produce inaccurate results. When predictions are inaccurate, valuable care manager resources are wasted on patients who don’t need the assistance, and the selection criteria becomes at risk of socio-economic and racial bias. Though these shortcomings can be adjusted by care managers, it results in another manual process — a step backward.
The Business Solution
Comprehensive Learning Algorithms
Modern AI techniques have the potential to solve these problems, unlocking advanced automation while accurately identifying patients in need of help. Recent advancements in deep learning have greatly expanded the tool set available to characterize a patient’s current health, building a more complete and accurate representation to forecast the right patient’s future needs at the right time.
Instead of requiring data to be distilled into limited categories and inflexible hierarchies, modern AI models use the full health record of the patient, tracking contextual relationships between all health events, sequencing and timing. State-of-the-art natural language processing techniques can also understand and utilize a physician’s written notes, which document influential details outside of a patient’s diagnosis or procedure codes.
To understand patients from a holistic perspective, it’s key to assess data beyond claims and billing history. Social determinants of health, including a patient’s physical environment, income, access to care and more, heavily influence the effectiveness of intervention.
Seeing the Whole Picture – Social Determinants of Health
80% of a person’s health is impacted by non-clinical factors.³
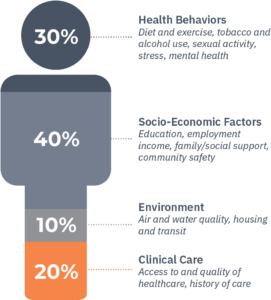
- Magnan, S. 2017. Social Determinants of Health 101 for Health Care: Five Plus Five. NAM Perspectives. Discussion Paper, National Academy of Medicine, Washington, DC. https://doi.org/10.31478/201710c
The Industry Impact
Learning Algorithms Applied
While working as an applied research scientist driving AI initiatives for a leading health payer, my team and I were faced with several challenges building a technology that had both an effective use case and business case. The organization had an existing goal of reducing patient readmissions, and due to the sheer amount of chronic patient data available, we discovered a deep learning framework could be an excellent tool to predict readmission.
Leveraging this data and a series of advanced techniques, we built a deep learning framework that was later applied to various functions within the care management lifecycle. We were able to efficiently build a model to risk score patients and determine those at highest risk for outreach in real time. This program allowed care managers to intervene while patients were still in the hospital, effectively reducing 30-day readmissions by 39%.
The same framework was successfully adapted to identify and engage members for chronic disease management. The framework was customized to predict a set of disease-specific preventable outcomes early, allowing enough time for the care manager to effectively intervene.
Eliminating the "Black Box"
Since each model was built independently of the others, we were able to directly incorporate feedback from care managers and clinical staff during the development process, tailoring the model to each preventable outcome. We wanted to ensure the care team provided direct input and quickly saw improvements, which built trust in the model and aligned the model with their needs.
To gather effective feedback, we developed tools and techniques to describe how the model operated in ways the care team would understand, eliminating the “black box” stigma commonly associated with these advanced methods.
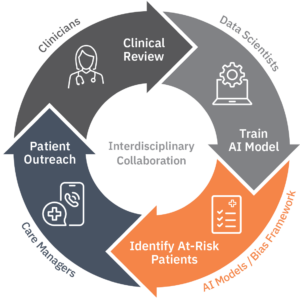
This process also allowed us to review the framework for socio-economic disparities and correct any bias in the system as it arose, enabling us to adjust before it could have a disproportionate impact on the people we were trying to help. The platform underwent weekly improvements, all of which were informed by care manager input and various harmful bias assessments by our internal medical council. By including feedback as a normal part of development and monitoring, the model was well prepared to be leveraged and standardized across the entire organization.
The Business Outcome
Once the platform launched, we saw patient engagement increase to 55%, efficiency increase by over 20% and a reduction in care manager workloads by an impressive 40%. We also discovered our organization became twice as effective while reducing costs. Leveraging the model as a tool, care managers were able to catch patterns in chronic patient care earlier and with more accuracy, resulting in over $40 million saved in medical expenses.
The AI model enabled clinical teams to target the right patient at the right time, earlier and with more accuracy.⁴
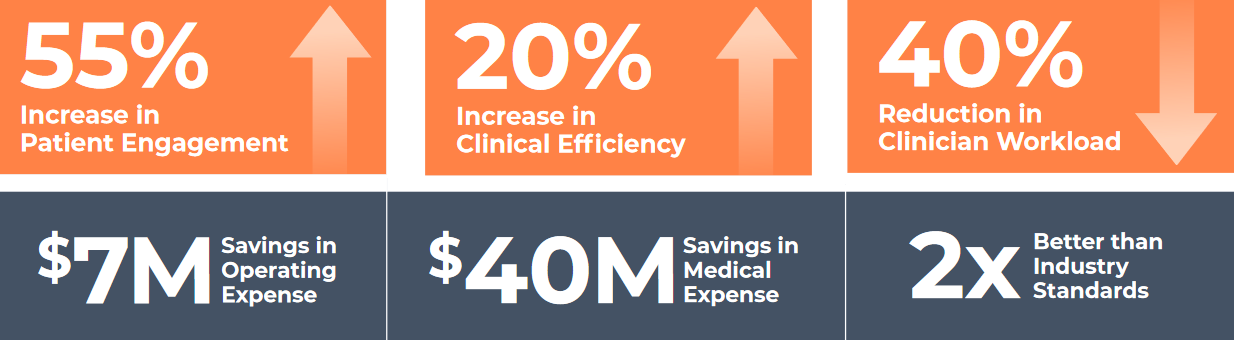
- The AI model predicts readmissions with much better accuracy, compared to previous in house model (retrospective markers) and compared to industry benchmark (LACE). Resulted in $7M Operating Savings, over $40M Medical Expense Savings, and improvements in Engagement Success Rates across Transitions of Care and Case Management. Transitions of Care engagement up from 12% to 57%. Case Management engagement up from 40% to 55%
Conclusion
Workflow and process inefficiencies are not isolated to chronic care management, plaguing countless verticals across the healthcare industry. Our deep learning framework opened the door for other AI use cases within healthcare, including finance or complex case management, which are currently being explored and tested.
AI isn’t a singular investment — it unlocks organization-wide potential. I am excited to continue this journey, as the possibilities of this technology has not been fully realized. If we, as an industry, continue approaching innovative projects with the right scope and goal in mind, we will see AI transform patient and clinical outcomes for the better.